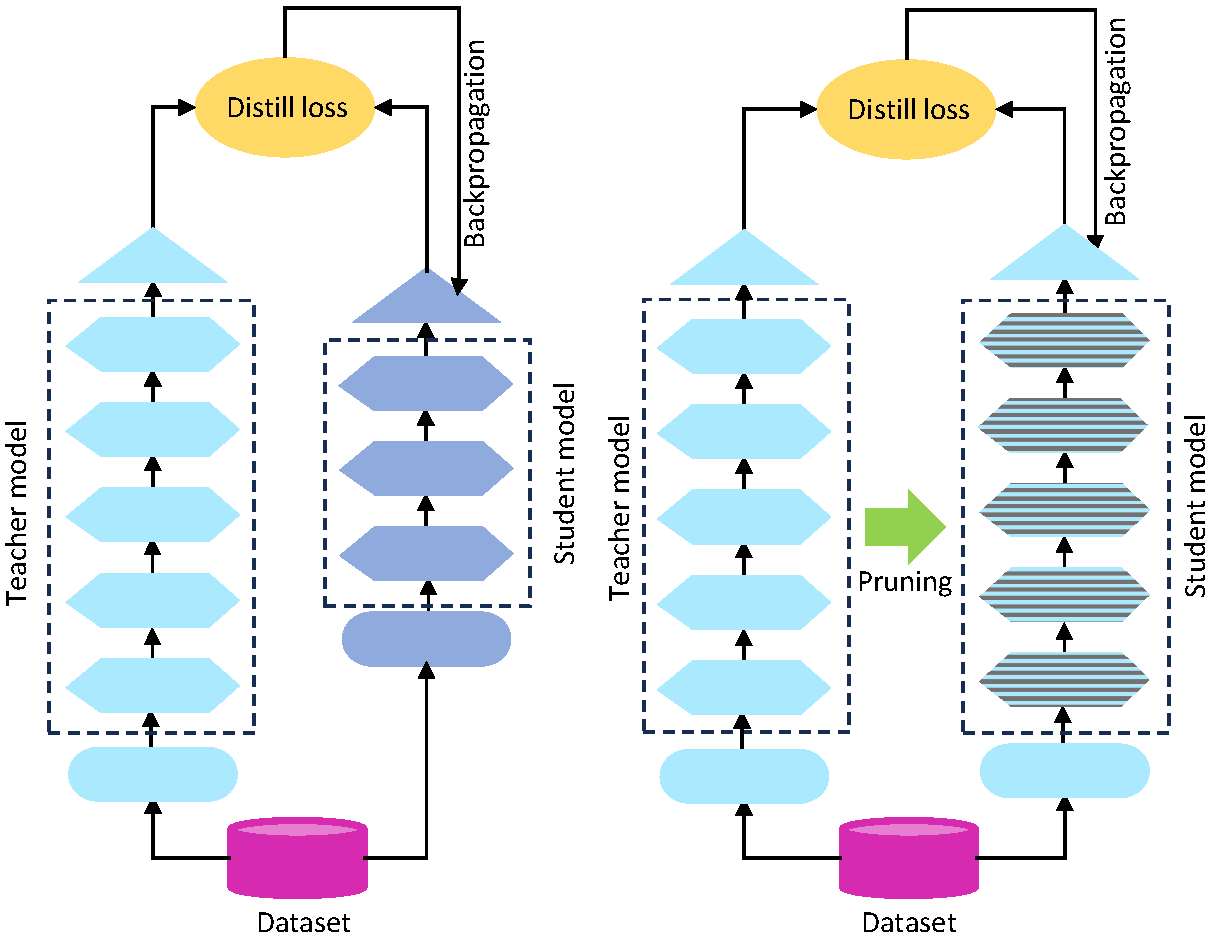
Azhar Shaikh, Michael Cochez, Denis Diachkov, Michiel de Rijcke, Sahar Yousefi, Want@NeurIPS 2023
Abstract: This paper introduces DONUT-hole, a sparse OCR-free visual document under- standing (VDU) model that addresses the limitations of its predecessor model, dubbed DONUT. The DONUT model, leveraging a transformer architecture, over- coming the challenges of separate optical character recognition (OCR) and visual semantic understanding (VSU) components. However, its deployment in produc- tion environments and edge devices is hindered by high memory and computational demands, particularly in large-scale request services. To overcome these challenges, we propose an optimization strategy based on knowledge distillation and model pruning. Our paradigm to produce DONUT-hole, reduces the model denisty by 54% while preserving performance. We also achieve a global representational similarity index between DONUT and DONUT-hole based on centered kernel alignment (CKA) metric of 0.79. Moreover, we evaluate the effectiveness of DONUT-hole in the document image key information extraction (KIE) task, highlighting its potential for developing more efficient VDU systems for logistic companies.